Reinforcement Learning (RL): how robots learn from their environment
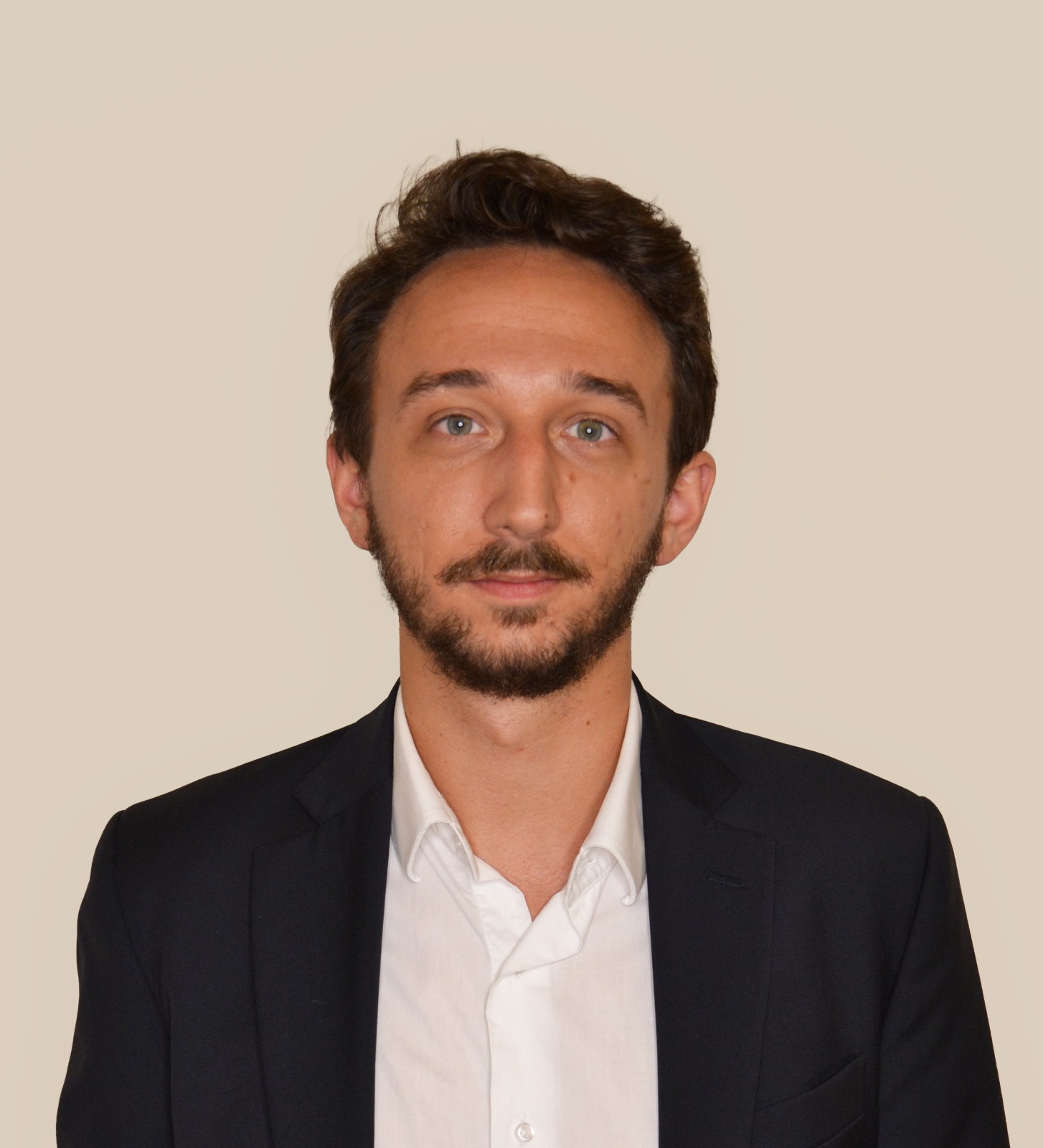
Piercosma Bisconti is co-funder of DEXAI – Artificial Ethics, a research company addressing the ethical and social challenges of Artificial Intelligence and robotics, with a particular interest in relational technologies and social robotics. He received his PhD in Political Philosophy from the Sant’Anna School of Advanced Studies in Pisa with a dissertation entitled “Socio-Technical Hybrid Societies: A Systemic Approach to Human-Machine Interactions.” Go to profile